Translate this page into:
Artificial intelligence in cancer pathology: Applications, challenges, and future directions

Dongmei Shi
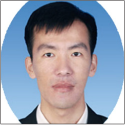
Biao Chen
*Corresponding author: Dongmei Shi, Laboratory of Medical Mycology, Jining No.1 People’s Hospital, Jining, Shandong, China. shidongmei28@163.com
Biao Chen, Central Laboratory, Jining key laboratory for the intelligent diagnosis of emerging infectious diseases, Jining No.1 People’s Hospital, Jining, Shandong, China. cbjn@mail.jnmc.edu.cn
-
Received: ,
Accepted: ,
How to cite this article: Wang J, Wang T, Han R, Shi D, Chen B. Artificial intelligence in cancer pathology: Applications, challenges, and future directions. CytoJournal. 2025;22:45. doi: 10.25259/Cytojournal_272_2024
Abstract
The application of artificial intelligence (AI) in cancer pathology has shown significant potential to enhance diagnostic accuracy, streamline workflows, and support precision oncology. This review examines the current applications of AI across various cancer types, including breast, lung, prostate, and colorectal cancer, where AI aids in tissue classification, mutation detection, and prognostic predictions. The key technologies driving these advancements include machine learning, deep learning, and computer vision, which enable automated analysis of histopathological images and multi-modal data integration. Despite these promising developments, challenges persist, including ensuring data privacy, improving model interpretability, and meeting regulatory standards. Furthermore, this review explores future directions in AI-driven cancer pathology, including real-time diagnostics, explainable AI, and global accessibility, emphasizing the importance of collaboration between AI and pathologists. Addressing these challenges and leveraging AI’s full potential could lead to a more efficient, equitable, and personalized approach to cancer care.
Keywords
Artificial intelligence
Cancer pathology
Deep learning
Global accessibility
Machine learning
INTRODUCTION
The rapid advancement of artificial intelligence (AI) has had a transformative impact across many sectors, and its application in cancer pathology is particularly notable. Cancer remains one of the leading causes of death worldwide, with millions of new cases diagnosed each year. Pathology, especially histopathology, plays a central role in the diagnosis and classification of cancer by examining tissue samples to identify malignant cells and structures. However, traditional pathology is a labor-intensive and time-consuming process that can be prone to subjective interpretation and diagnostic variability between pathologists. The global shortage of skilled pathologists is a pressing issue, particularly in low- and middle-income countries. Research published in The Lancet points to a deficit of more than 1 million pathologists worldwide, with many countries having fewer than two pathologists per million people.[1] In the United States, there is an estimated shortage of 14,000 pathologists by 2030, and in rural regions, the shortage can be even more severe.[2]
AI, particularly deep learning (DL) and machine learning (ML) has emerged as a powerful tool to address these challenges in cancer pathology.[3] AI can analyze vast amounts of data, including complex medical images, with greater speed and consistency than traditional manual methods.[4] AI-driven systems are capable of detecting, segmenting, and classifying tumor cells with impressive accuracy, often matching or surpassing the performance of human pathologists in certain tasks.[5] This makes AI an invaluable tool for improving diagnostic accuracy, reducing intra- and inter-observer variability, and enhancing the efficiency of pathology workflows.
AI is not only transforming cancer diagnosis but also contributing to personalized medicine by predicting treatment responses and patient outcomes based on a combination of histopathological, genomic, and clinical data.[6] These AI-driven advancements are paving the way for more individualized, precise cancer treatment plans, which are essential in improving patient survival rates and quality of life.[7] However, the integration of AI into clinical practice is not without its challenges, such as the need for large, well-annotated datasets, ensuring model interpretability, and addressing regulatory and ethical concerns. This article aims to systematically summarize the various opportunities and challenges associated with AI in cancer pathology by providing insights into the current limitations, ethical considerations, and future directions, offering guidance for both researchers and practitioners in the field.
OVERVIEW OF AI APPLICATIONS IN CANCER PATHOLOGY
AI has gained significant traction in cancer pathology due to its ability to process vast amounts of data with remarkable accuracy.[5] Core AI technologies, such as ML, DL, and computer vision, have been particularly transformative in the analysis of histopathological images. By utilizing convolutional neural networks (CNNs), AI can automatically detect, segment, and classify cancerous tissues, reducing the dependency on manual interpretation by pathologists. One of the primary advantages of AI in pathology is its ability to minimize human error and intra-observer variability, offering consistent and reproducible results.[3] In addition, AI can significantly expedite the diagnostic process, enabling real-time or near-real-time analysis that shortens the turnaround time for pathology reports. Moreover, AI algorithms can integrate multiple data types, such as clinical and genomic data, alongside pathology images to provide comprehensive insights into tumor behavior, aiding in precision medicine. While AI is not intended to replace pathologists, it can serve as a valuable tool for augmenting their expertise and reducing their workload in the face of rising cancer cases globally.[8] A workflow depicting the integration of AI in cancer pathology is shown in Figure 1.
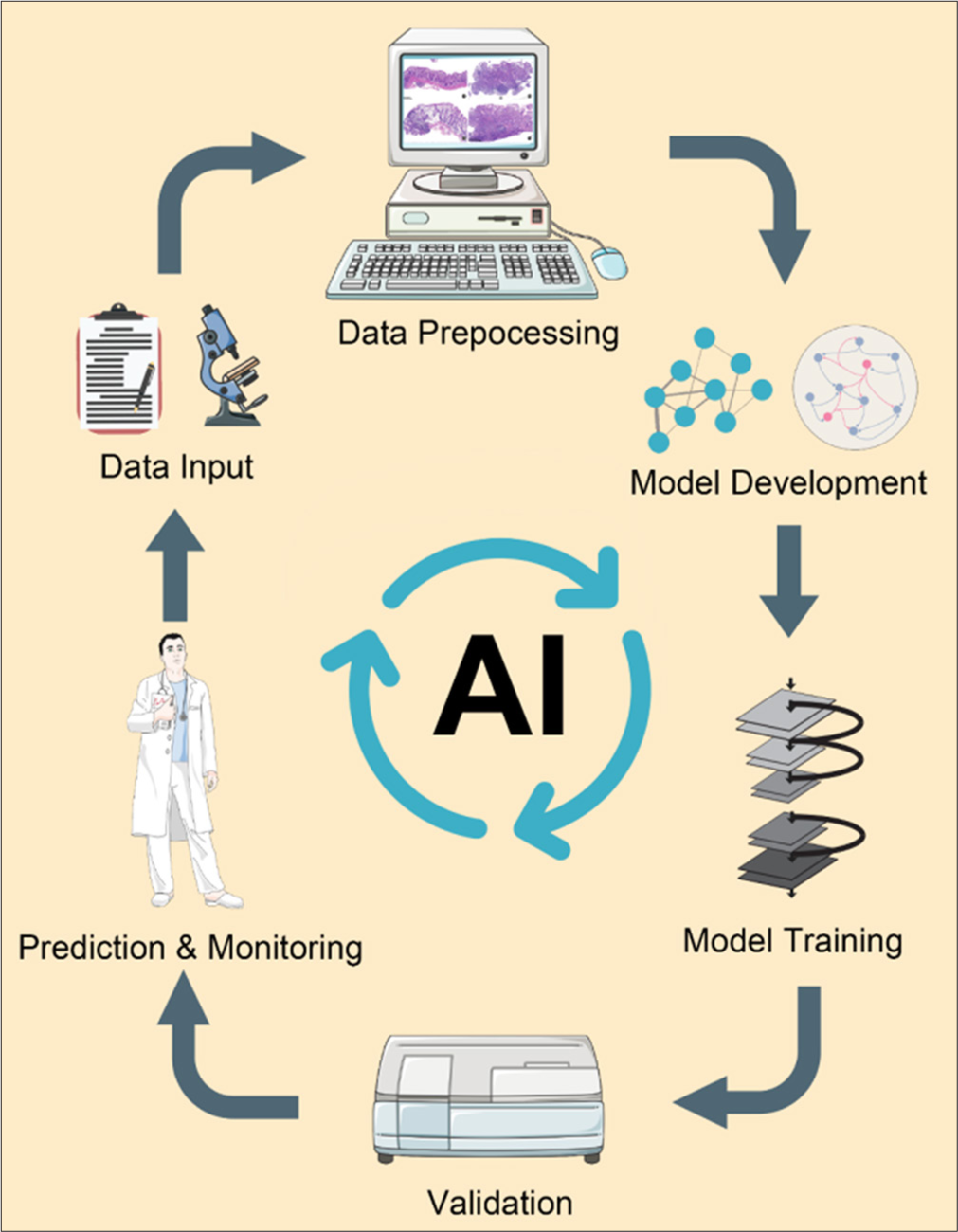
- A workflow of artificial intelligence (AI) in cancer pathology (Name: Adobe After Effects; Version: CC 2023; Manufacturer: Adobe Systems Origin: United States/Name: Adobe Photoshop; Version: 2023.25; Manufacturer: Adobe Systems; Origin: United States; the URL of the database referenced by all elements: https://smart.servier.com/ and https://scidraw.io/. These platforms provide materials under the CC BY 4.0 license, which permits free use with proper attribution.).
Comparison with traditional methods in cancer pathology
To recognize the remarkable potential of AI in cancer pathology, it is essential to highlight how it addresses some of the limitations inherent in traditional methods. Traditional cancer diagnosis and staging rely heavily on manual examination of histopathological slides by pathologists, a process that can be time-consuming, subjective, and prone to human error.[9] As discussed in the following sections, AI offers several solutions to these issues, enhancing diagnostic accuracy, efficiency, and scalability.[10]
Increased diagnostic speed
Traditional methods often require pathologists to manually examine thousands of tissue samples, which can result in long turnaround times for cancer diagnosis.[11] AI systems, however, can analyze large volumes of medical images in a fraction of the time, significantly speeding up the diagnostic process.[12] For example, AI algorithms have been shown to reduce the time needed for tumor detection in breast cancer and melanoma compared to manual analysis, allowing for quicker treatment decisions and potentially better patient outcomes.[10,12]
Enhanced diagnostic accuracy
Pathologist interpretation can be affected by fatigue, experience, and the complexity of the tissue samples.[13] AI can assist in overcoming these limitations by providing consistent and unbiased results.[14] Studies have shown that DL algorithms can outperform human pathologists in certain cancer detection tasks, such as detecting lymph node metastasis in breast cancer or identifying early-stage lung cancer lesions.[10,12] This consistency ensures that diagnoses are less likely to be missed or misinterpreted, particularly in difficult cases or rare cancer types.
Reduction of inter-observer variability
In traditional pathology, the interpretation of slides can vary between different pathologists, leading to inconsistent results.[15] This inter-observer variability is particularly problematic in cancer grading, where slight differences in judgment can affect treatment decisions. AI can help standardize diagnoses by providing an objective analysis of tissue samples.[16] For instance, AI models used in prostate cancer grading have demonstrated greater consistency than human pathologists in assessing Gleason scores, which are crucial for determining the cancer’s aggressiveness and treatment options.[17]
Integration with multi-modal data
Unlike traditional methods that primarily focus on histopathological images, AI systems can integrate various forms of patient data, such as genomic sequences, clinical histories, and radiological images, to provide a more comprehensive view of the patient’s condition.[18] This multi-modal approach allows for more personalized treatment plans and better-informed clinical decisions. For example, AI models that integrate genetic data with pathology slides can help identify patients who are most likely to benefit from targeted therapies or immunotherapy, something that is difficult to achieve using traditional methods alone.[19]
Enhanced access to diagnostics in underserved areas
Traditional pathology services often face shortages, especially in rural or low-resource settings, leading to delays in cancer diagnosis and treatment. AI-driven digital pathology platforms and telepathology can overcome these barriers by enabling remote diagnostic capabilities. Pathologists can collaborate with AI tools to analyze digital slides from anywhere in the world, improving access to timely and accurate diagnoses even in regions lacking specialized expertise.[16,20]
AI APPLICATIONS IN SPECIFIC CANCER TYPES
AI has made substantial contributions to cancer pathology by providing automated and highly accurate diagnostic tools tailored to different types of cancers. Each cancer type poses unique challenges, and AI has been adapted to address these needs, leading to a variety of tailored applications across various malignancies. Applications of AI in cancer pathology are shown in Figure 2.
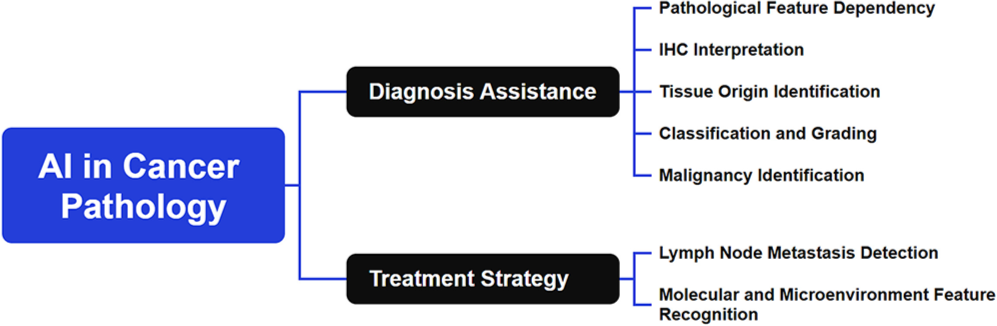
- Applications of artificial intelligence in cancer pathology (Name: Xmind; Version: Xmind 15.0; Manufacturer: BeijingZhitu; Origin: China). Note: IHC: Immunohistochemistry.
Breast cancer
Breast cancer is one of the most studied areas for AI applications in pathology. AI algorithms have shown remarkable capability in analyzing whole-slide imaging (WSI) datasets to identify and grade invasive ductal carcinoma and ductal carcinoma in situ. DL models, such as CNNs, have been employed to automate human epidermal growth factor receptor 2 (HER2) scoring from immunohistochemistry slides, achieving performance comparable to expert pathologists in classifying HER2 status, which is crucial for treatment decisions.[21,22] Furthermore, AI has been used to predict Oncotype DX scores directly from pathology images, providing an alternative to expensive gene expression tests.[6] By automating processes, AI improves workflow efficiency, providing more reliable diagnoses that lead to better patient outcomes. An example of estrogen receptor quantification by an AI algorithm is shown in Figure 3.[23]
![Example of estrogen receptor quantification by artificial intelligence algorithm.[23]](/content/105/2025/22/1/img/Cytojournal-22-45-g003.png)
- Example of estrogen receptor quantification by artificial intelligence algorithm.[23]
Lung cancer
Lung cancer, which includes several histological subtypes, poses a significant diagnostic challenge. AI has been utilized to distinguish between small cell lung carcinoma (SCLC) and non-small-cell lung carcinoma (NSCLC) based on complex morphological patterns.[24] Cai et al. developed a DL model that successfully differentiated between SCLC and NSCLC using histopathological images, achieving an accuracy of 91% that outperformed traditional diagnostic methods.[25] AI-based systems have shown potential in identifying specific mutations, such as estimated glomerular filtration rate (EGFR) and anaplastic lymphoma kinase alterations directly from histopathological images, which could guide targeted therapy decisions.[26,27] Mahajan et al. demonstrated that AI-based models could identify EGFR mutations from tissue samples with an accuracy of 88%, providing valuable insights for personalized treatment decisions in lung cancer patients.[28] In early-stage lung cancer, AI-based radiomics and pathology integration models have been used to predict lymph node metastasis and patient prognosis, enhancing decision-making for surgical interventions.[29,30] These applications show that AI aids in the accurate differentiation between cancer subtypes, which is vital for tailored treatment planning. By identifying genetic mutations and predicting metastasis, AI supports earlier and more precise interventions, improving overall patient prognosis.
Prostate cancer
Prostate cancer diagnosis and grading have traditionally been highly subjective, leading to inter-observer variability among pathologists. AI has been shown to reduce this variability by providing automated Gleason grading from prostate biopsy images.[31] AI models, such as those developed by Arvaniti et al., can distinguish between benign and malignant glands with high sensitivity and specificity and even predict biochemical recurrence based on histological features alone.[32] A study demonstrated the application of DL to prostate cancer histopathology slides, achieving high performance in Gleason grading and tumor classification, outperforming pathologists in certain cases.[33] Likewise, a study included a DL algorithm that automatically grades prostate cancer from pathology slides, significantly reducing inter-observer variability and improving diagnostic consistency.[34] This level of detail supports more accurate prognostication and risk stratification in prostate cancer management. AI reduces grading variability, which is critical for accurate risk stratification. By automating Gleason grading, AI ensures more reliable results, which aids personalized treatment decisions, leading to better prognostic outcomes.
Colorectal cancer (CRC)
AI applications in CRC have focused on tumor segmentation, detection of tumor budding, and prediction of lymphovascular invasion. Automated systems have been developed to identify microsatellite instability (MSI) from standard histology slides, providing a cheaper alternative to molecular testing.[6] For instance, Qiu et al. used DL models to detect MSI directly from hematoxylin and eosin (H&E) stained slides, achieving accuracy comparable to traditional molecular methods.[35] Moreover, DL models have shown high accuracy in detecting tumor-infiltrating lymphocytes (TILs), which are important prognostic markers for CRC.[3] In a study by Song et al., AI demonstrated strong performance in classifying tumor budding, which is critical for determining patient prognosis and treatment planning.[36] AI has also been used to differentiate between adenomatous and hyperplastic polyps in endoscopic biopsy samples, aiding early detection and prevention.[22] By identifying TILs and MSI, AI enhances the accuracy of CRC diagnosis and prognosis. This helps in early detection and prevention, reducing unnecessary procedures and improving treatment outcomes.
Skin cancer
Skin cancer, particularly melanoma, is one of the most lethal forms of cancer if not diagnosed early. AI has demonstrated dermatologist-level accuracy in classifying skin lesions from histopathological images, especially for melanomas and basal cell carcinomas. Esteva et al. employed a deep neural network to achieve performance on par with dermatopathologists in identifying skin cancers from biopsy images.[10] In addition, AI has been used to evaluate Breslow thickness and predict lymph node involvement, which are critical factors in melanoma staging and management.[37] Gillstedt et al. developed a DL model that accurately predicts Breslow thickness from dermoscopic images, which is vital for assessing melanoma prognosis and treatment options.[38] Moreover, Xu et al. used AI to assess lymph node involvement in melanoma patients, showing that AI models can predict metastatic risk, thus aiding in personalized treatment planning.[39] By achieving dermatologist-level accuracy, AI plays a pivotal role in early skin cancer detection, improving survival rates. Through its ability to predict lymph node involvement and assess Breslow thickness, AI also helps in personalized treatment planning for melanoma patients.
Gastrointestinal cancers (gastric and esophageal cancer)
Gastric and esophageal cancers are often diagnosed at advanced stages due to subtle histopathological changes in early disease. AI has been applied to detect early gastric cancer from biopsy samples, achieving high sensitivity even in cases with minimal morphological alterations.[40-42] Similarly, AI has been used to predict HER2 expression status in gastric cancer from routine H&E slides, assisting in the selection of targeted therapies.[43] For esophageal cancer, AI systems have been developed to assess tumor regression grades after neoadjuvant therapy, providing insight into treatment efficacy.[44-46]AI enhances early-stage detection of gastric cancer, which is vital for improving survival rates. By predicting HER2 expression status and evaluating treatment response, AI further aids in the selection of appropriate therapies, making it an invaluable tool for personalized cancer care.
Brain cancer
AI has shown promise in the diagnosis and grading of gliomas, which are among the most aggressive forms of brain cancer. DL models have been applied to segment and classify tumor regions from histopathological images, outperforming human experts in some scenarios.[16,47-50] In addition, AI-based approaches have been used to identify key genetic mutations (e.g., Isocitrate Dehydrogenase 1/2 status) and predict overall survival, offering comprehensive tools for personalized management of gliomas.[3] A DL-based survival prediction model that incorporated radiological and histopathological data provided a more accurate prognosis for glioma patients than traditional methods.[51] The above evidence indicates that AI’s application in glioma diagnosis enhances the accuracy of tumor grading and genetic profiling, which helps in devising more personalized and effective treatment strategies. By predicting survival and identifying mutations, AI optimizes treatment planning and patient management for glioma cases.
These applications highlight how AI is revolutionizing cancer pathology by providing reliable, reproducible, and clinically actionable insights across various cancer types. As these technologies continue to evolve, they have the potential to fundamentally change how cancer is diagnosed and managed, ultimately improving patient outcomes.[50]
KEY CAPABILITIES AND TECHNIQUES OF AI IN CANCER PATHOLOGY
AI in cancer pathology relies on several advanced technological developments that drive its ability to perform complex image analysis, classification, and prediction. These technologies provide the foundation for automating traditionally manual processes and offer pathologists new tools to enhance diagnostic precision.
Image segmentation and classification
One of the key components of AI applications in pathology is the ability to segment and classify histopathological images accurately. CNNs have become the primary architecture for these tasks due to their ability to capture hierarchical spatial features in images. In cancer pathology, CNN-based models have been applied to detect tumor boundaries, differentiate between cancerous and non-cancerous tissues, and grade tumor malignancies.[5,24] The challenge, however, lies in achieving fine-grained segmentation at cellular or sub-cellular levels, especially in densely packed tissue regions. Solutions such as U-Net and Mask R-CNN have been specifically designed for medical image segmentation, enabling the precise delineation of tumor areas in complex tissue environments.[52]
Super-resolution and image enhancement
AI algorithms also contribute to improving the quality of low-resolution pathology images. Super-resolution techniques have been applied to enhance images acquired from lower magnification, enabling detailed analysis without the need for high-resolution scanning, which can be time-consuming and resource-intensive.[53-56] These approaches are particularly useful for large-scale screening tasks where high-resolution images are not always necessary, but accurate detection is still required.
Data annotation and labeling
Training AI models for cancer pathology requires large, well-annotated datasets. This presents a major challenge, as manually labeling histopathological images is labor-intensive and requires expert knowledge. To address this, AI tools are being developed to assist with semi-automated annotation, allowing pathologists to mark regions of interest more efficiently. In addition, active learning techniques are employed to iteratively improve the AI model by selectively querying new data points for labeling.[57,58] This reduces the amount of data that needs to be manually annotated while still improving the model’s performance.
Handling large-scale data and high-throughput analysis
One of the primary advantages of AI in cancer pathology is its ability to handle large-scale datasets. WSI, which involves scanning entire pathology slides at high resolution, generates enormous amounts of data. DL models continue to be developed to process these large images efficiently by dividing them into smaller tiles for analysis.[59] Techniques like tile-based training, along with cloud computing infrastructure, have enabled high-throughput analysis that can assist pathologists in rapidly identifying areas of concern across thousands of images.
Training, validation, and model generalization
A significant challenge in the application of AI is ensuring that models generalize well across different datasets and populations. Pathology images can vary greatly due to differences in tissue preparation, staining protocols, and scanner equipment. To mitigate this issue, transfer learning has been adopted, where a pre-trained model is fine-tuned on a smaller, task-specific dataset, improving its performance with limited data.[60] Furthermore, techniques such as data augmentation (random rotations, flipping, or color jittering) are used to artificially expand the training dataset and improve model robustness.[61]
Model validation and interpretation
Once trained, AI models must be thoroughly validated before clinical implementation. This often involves multi-institutional validation to test the model’s generalization capabilities across different patient populations and clinical settings.[62] Explainability is another critical factor in ensuring the adoption of AI in clinical practice. Although DL models, particularly CNNs, are often seen as “black boxes,” efforts are underway to make them more interpretable. Techniques such as Gradient-weighted Class Activation Mapping (Grad-CAM) allow pathologists to visualize which regions of the image contributed most to the model’s prediction, fostering trust in AI-generated results.[63]
Integration with multi-modal data
AI models are increasingly being designed to integrate histopathological data with other clinical information, such as genomic data and patient histories, to provide a more comprehensive diagnostic picture. This multi-modal approach helps refine predictions related to cancer prognosis, treatment response, and patient survival.[64] The use of multi-modal AI models in cancer pathology represents a shift toward more personalized medicine, where treatment decisions are based not only on pathology images but also on a wide range of patient-specific data.
These technological advancements underscore the complexity of AI applications in cancer pathology. While these models offer considerable potential, ensuring their reliable performance across diverse datasets and making them interpretable for clinicians are key areas that continue to evolve.
CHALLENGES OF AI IN CANCER PATHOLOGY
Despite the remarkable advancements of AI in cancer pathology, there are significant challenges that must be addressed before it can be fully integrated into clinical practice. These challenges span across various dimensions, including data-related issues, algorithm reliability, ethical concerns, and the practical collaboration between AI systems and pathologists. The main challenges and potential solutions of AI application in cancer pathology are presented in Figure 4.
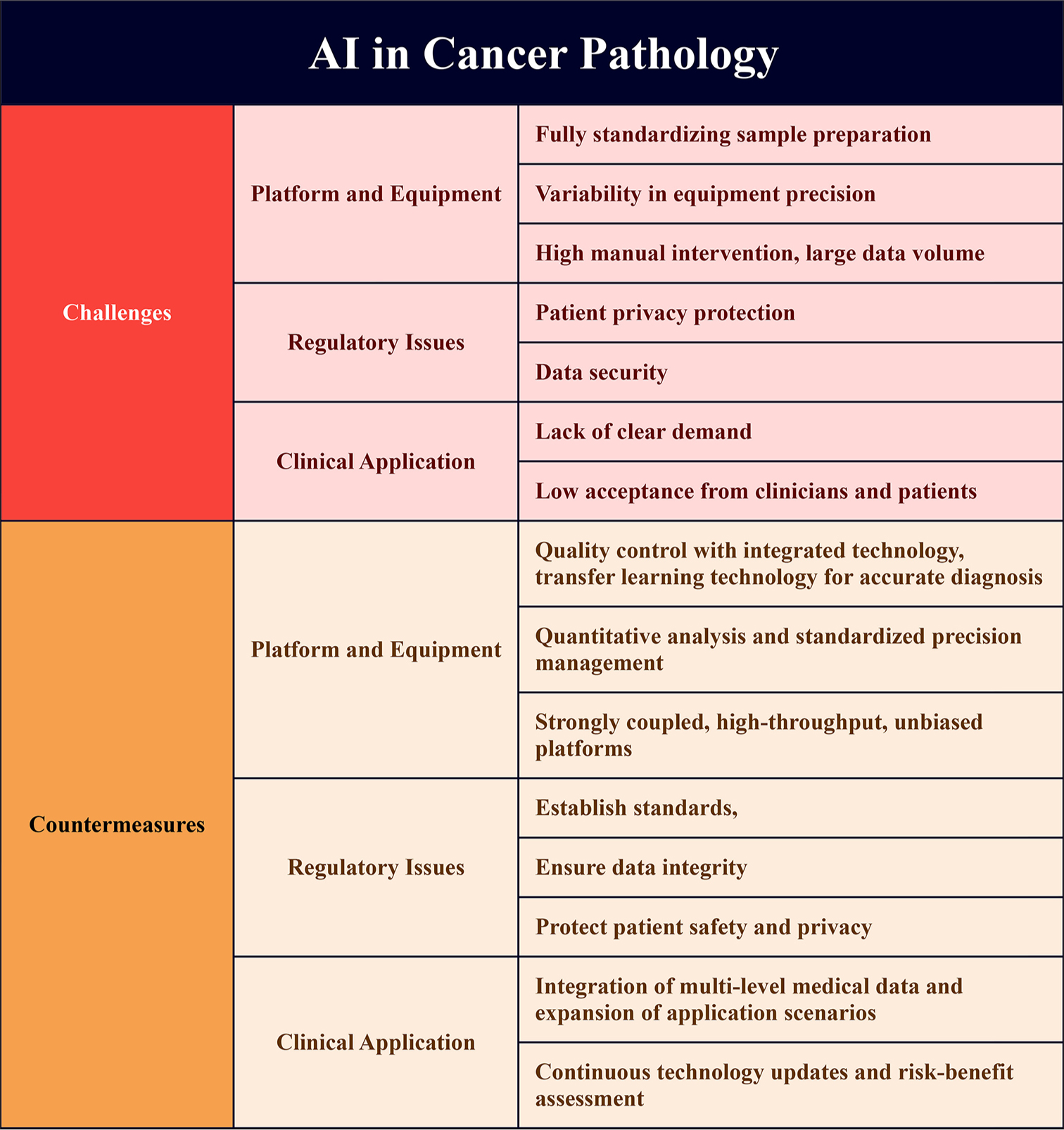
- Challenges and solutions of artificial intelligence application in cancer pathology. (Name: Xmind; Version: Xmind 15.0; Manufacturer: BeijingZhitu; Origin: China).
Data privacy and security
One of the most critical concerns in AI-driven pathology is the security and privacy of patient data. Cancer pathology requires the use of vast amounts of sensitive, personally identifiable information, including histopathological images, genomic data, and clinical records. The widespread adoption of AI tools necessitates secure storage and transmission of this data, ensuring compliance with regulations such as the Health Insurance Portability and Accountability Act in the United States and the General Data Protection Regulation in Europe.[10] Balancing the need for large, diverse datasets to train robust AI models with stringent privacy standards remains a key challenge. Secure data-sharing frameworks and anonymization techniques are, therefore, essential for enabling multi-institutional collaboration without compromising patient confidentiality.[65]
Algorithm reliability and generalization
AI algorithms, particularly DL models, can be highly sensitive to variations in data, such as differences in slide preparation, staining protocols, and imaging equipment across different laboratories.[3] This lack of standardization can lead to performance discrepancies when AI models are deployed in real-world clinical settings. Moreover, AI systems are prone to overfitting when trained on limited or highly specific datasets, meaning they may perform well on the data they were trained on but fail to generalize to new, unseen cases.[66] Addressing these issues requires larger, more diverse datasets and rigorous external validation across multiple institutions to ensure that AI models are robust and reliable in varied environments.
Interpretability and the “Black Box” problem
DL models, while effective, often operate as “black boxes” that produce predictions without offering clear insights into their decision-making processes. This lack of interpretability is a significant barrier to clinical adoption, as pathologists and clinicians need to understand how an AI system arrives at its conclusions, especially in high-stakes decisions such as cancer diagnosis.[67] Techniques such as Layer-wise Relevance Propagation and SHapley Additive exPlanations (SHAP) are being developed to improve the transparency of AI models by highlighting which parts of the input data are most influential in the model’s predictions.[68] However, these methods are still evolving, and achieving full transparency remains an ongoing challenge in AI-driven cancer pathology.
Regulatory and legal issues
The integration of AI into clinical practice presents profound legal and regulatory challenges that need careful consideration. In many jurisdictions, medical devices, including AI software used for diagnostic purposes, must undergo regulatory approval to ensure they meet stringent safety, efficacy, and reliability standards before being used in clinical settings. However, the dynamic nature of AI models complicates this process. Unlike traditional medical devices, which are typically static once approved, AI systems are often updated or retrained based on new data, making it difficult to maintain consistent regulatory oversight.[69] This evolution in AI systems means that their performance can change over time, raising concerns about how regulators can ensure ongoing compliance with safety standards. Moreover, the legal ramifications of AI errors or misdiagnoses remain ambiguous. In the event of an incorrect diagnosis made by an AI system, questions of liability arise – should the responsibility lie with the software developer, the healthcare provider who relied on the system, or the institution that deployed it? This legal gray area can hinder the widespread adoption of AI technologies, as healthcare providers and developers may be hesitant to embrace these tools without clear guidelines. The creation of robust regulatory frameworks, accompanied by ethical guidelines that address issues like accountability, transparency, and patient consent, will be essential to ensure that AI is implemented safely and responsibly in cancer pathology. Only through such frameworks can the legal, ethical, and practical challenges of AI integration be effectively navigated, fostering greater confidence in its potential to enhance clinical practice without compromising patient safety.[70]
Ethical considerations and bias
Ethical issues are also a major concern in AI applications for cancer pathology.
Bias in AI models can arise from imbalanced or historically biased training datasets, which may reflect disparities in previous clinical practices or underrepresentation of certain demographics. For example, the underrepresentation of minority groups in training data can result in algorithms that perform poorly for those populations, perpetuating healthcare inequities.[71] To mitigate these concerns, AI developers have an ethical responsibility to ensure fairness and equity using diverse and inclusive datasets, which are crucial for enhancing the generalizability of AI models across all patient groups.
Furthermore, the relationship between AI and pathologists raises important ethical considerations. While AI can reduce human error and improve diagnostic speed, it should complement, not replace, human expertise. Ethical guidelines should emphasize that AI is an augmentation tool – empowering pathologists to make better decisions – but not a substitute for human judgment in complex or ambiguous cases. In addition, issues of accountability and patient consent must be carefully addressed, especially when communicating AI-generated diagnoses and recommendations to patients.[72]
Collaboration between AI and pathologists
While AI has the potential to revolutionize cancer pathology, it must be emphasized that AI is not meant to replace human pathologists but to complement their expertise. AI systems can process vast amounts of data quickly and consistently, but pathologists bring contextual understanding and clinical judgment that AI models cannot replicate. The challenge lies in integrating AI into the clinical workflow in a way that enhances the efficiency and accuracy of pathology without diminishing the role of the human pathologist.[31] Developing user-friendly AI tools that pathologists can trust and collaborate with is essential for successful implementation.
Cost and infrastructure
Finally, the implementation of AI in cancer pathology requires significant investments in digital infrastructure, training, and maintenance. Pathology labs must adopt WSI technology, cloud storage solutions, and high-performance computing systems to support AI algorithms.[3] For many healthcare institutions, particularly those in resource-limited settings, these costs may be prohibitive. In addition, training pathologists to work with AI tools and ensuring that these systems are maintained and updated regularly poses ongoing challenges. Reducing the cost of AI deployment and making these tools accessible to a wider range of healthcare providers is crucial for the widespread adoption of AI in cancer pathology.
Risks of misapplication of AI in cancer pathology
While AI holds great potential for advancing cancer pathology, there are risks associated with its misapplication.[73] One of the most concerning issues is the possibility of false diagnoses resulting from inaccurate AI predictions. This could lead to incorrect treatment plans, delays in diagnosis, or unnecessary treatments, all of which could compromise patient safety and outcomes. Over-reliance on AI systems is another potential danger, where pathologists may defer too heavily to AI suggestions, reducing human oversight and critical thinking. This could be particularly problematic in complex or rare cases where AI models may lack sufficient training data to make accurate predictions.[74]
To mitigate these risks, it is essential to maintain a collaborative approach between AI systems and human experts, ensuring that AI serves as a supportive tool rather than a replacement for clinical judgment. Ongoing monitoring, regular validation, and continuous education for pathologists on AI tools are key measures to prevent misapplications and maintain diagnostic quality.
These challenges highlight the complexity of integrating AI into cancer pathology. Addressing issues related to data privacy, algorithm reliability, model interpretability, regulatory frameworks, ethical considerations, and cost will be critical for the successful and responsible implementation of AI in clinical practice.
FUTURE DIRECTIONS AND PROSPECTS OF AI IN CANCER PATHOLOGY
The future of AI in cancer pathology holds significant promise, with ongoing developments expected to transform how cancer is diagnosed, treated, and managed. AI will continue to evolve, integrating more advanced technologies and addressing the current limitations to ensure its broader application and acceptance in clinical settings. Several key trends and future directions are likely to define the trajectory of AI in cancer pathology. Below are some key aspects that will shape the future of AI in this field.
Integration of multi-modal data
One of the most promising future directions for AI in cancer pathology is the integration of multi-modal data. As discussed previously, in addition to histopathological images, AI systems can incorporate clinical data, genomic sequencing, and radiological images to provide a more comprehensive understanding of cancer biology. By combining these data types, AI can offer more personalized and precise diagnostic and prognostic information, moving toward the ideal of precision oncology.[6,64] For example, AI models could link tumor morphology with genetic mutations, such as TP53 or KRAS, and suggest targeted therapies based on these molecular profiles. Multi-modal AI systems will likely play a crucial role in the early detection of cancers, prediction of treatment responses, and monitoring of disease progression.
Real-time AI-assisted diagnostics
The future of AI in cancer pathology is heading toward real-time diagnostics, where AI models can process pathology slides instantaneously during biopsies or surgeries. Such systems would allow pathologists to obtain AI-generated insights in real-time, assisting with intraoperative decision-making, such as determining clear surgical margins.[75,76] This rapid feedback loop could significantly reduce diagnostic turnaround times and improve surgical outcomes. Cloud-based solutions and edge computing, combined with AI, will enable real-time processing even in low-resource settings, making AI more accessible globally.
AI in early detection and cancer screening
Early cancer detection is a key factor in improving survival rates, and AI is poised to enhance cancer screening programs by detecting subtle patterns that may be missed by the human eye. AI models for screening are particularly promising in cancers where early-stage detection is difficult, such as ovarian, pancreatic, and lung cancers.[77] AI can be integrated with screening modalities such as mammography, computed tomography scans, and even blood-based liquid biopsies to detect cancer biomarkers at earlier stages than traditional methods.[18,78] These AI-powered screening tools could significantly improve early diagnosis and lead to better outcomes for cancer patients through earlier intervention.
Telepathology and remote diagnostics
Telepathology and AI-based remote diagnostics are poised to address the disparity in access to high-quality pathology services across the world, particularly in low-resource or rural areas. With AI-driven digital pathology platforms, pathologists can remotely analyze digitized slides and collaborate with AI tools to provide accurate diagnoses without being physically present.[79] As AI models become more robust, remote pathology services will be able to deliver quicker and more reliable results, bridging gaps in healthcare infrastructure. This approach has already gained momentum in some regions, and as digital pathology becomes more widespread, AI will enhance the efficiency of remote consultations.
Explainable AI (XAI) for greater trust and adoption
The development of XAI models is a critical future direction that will enhance trust in AI systems among clinicians and pathologists. XAI refers to AI models designed to make their decision-making processes transparent and interpretable, which is essential in clinical settings where human oversight is crucial. Pathologists need to understand why an AI model has made a particular recommendation or diagnosis to ensure its appropriateness for each patient. As XAI techniques such as Grad-CAM and SHAP improve, AI models will become more interpretable, providing visual explanations for decisions that align with human reasoning.[63,80] This transparency will be pivotal in increasing AI’s acceptance in pathology and ensuring patient safety.
Self-learning and continual learning AI models
AI models capable of self-learning or continual learning – adapting and evolving as new data become available – are another exciting area of future development. Traditional AI models are static once trained, but self-learning models can update themselves by learning from new cases or user feedback, improving their accuracy over time without needing full retraining from scratch.[81-83] In cancer pathology, this could lead to more adaptive systems that stay current with the latest clinical insights, evolving treatment protocols, and newly discovered biomarkers. These adaptive models would be highly valuable for rare cancers or newly emerging cancer subtypes where limited historical data are available.
AI-driven drug discovery and predictive modeling
Another major future direction is the use of AI in drug discovery and predictive modeling for cancer treatment. By analyzing pathology data, AI can help identify novel drug targets, predict patient responses to various treatments, and simulate the effects of new drugs. In oncology, AI models are being developed to predict which patients are likely to respond to immunotherapies based on the tumor microenvironment and genetic markers.[84] AI can also facilitate virtual clinical trials, where drug efficacy is tested on AI models before moving to human trials, significantly reducing costs and time in drug development.[85] These predictive tools will allow oncologists to personalize cancer treatment regimens, enhancing patient outcomes while reducing unnecessary treatments.[77]
Global application and resource distribution
A significant future prospect of AI in cancer pathology is its potential to democratize access to high-quality diagnostic services globally. Many developing countries and rural areas face a shortage of pathologists and diagnostic tools, which leads to delayed or inaccurate cancer diagnoses. AI-driven pathology systems can help bridge this gap by providing highly accurate, cost-effective, and scalable diagnostic solutions.[86] As AI systems improve in terms of cost, speed, and robustness, they could be deployed in resource-limited settings to improve cancer detection rates and provide equitable healthcare access worldwide.
Potential timeline and steps for achieving future directions
The realization of AI’s potential in cancer pathology will likely unfold in phases. In the short term, the focus will be on improving the accuracy and generalization of AI models for clinical applications, especially in real-time diagnostics and multi-modal data integration. During this phase, regulatory bodies will finalize guidelines and frameworks for AI adoption in clinical settings. In the medium term, AI tools will be more widely used in routine diagnostics and personalized treatment planning. Key developments will include enhanced data-sharing agreements and the creation of more robust, diverse datasets for AI training. Finally, in the long term, AI is expected to become integral to personalized oncology, with continuous learning models and broader deployment in global healthcare, particularly in underserved regions.[4,20]
Steps for achieving future goals
To achieve these goals, several steps are essential. First, regulatory support is crucial to establish clear standards for AI’s safety and efficacy in clinical practice. Second, data sharing across institutions and countries will be necessary to build diverse, representative datasets. In addition, continued advancements in technology development, especially in AI algorithms and computational power, will be critical. The success of AI integration into clinical workflows will also depend on education, ensuring healthcare professionals are trained to effectively work with AI tools. Finally, significant investment in infrastructure, particularly in low-resource settings, will be needed to facilitate the widespread adoption of AI technologies globally.[14,50]
These future directions point toward an increasingly collaborative relationship between AI and pathologists, where AI enhances diagnostic capabilities without replacing the human expert. By integrating AI into routine cancer diagnostics and care, the field of pathology will move toward more personalized, efficient, and accessible cancer management strategies.
DISCUSSION
The integration of AI in cancer pathology represents a significant paradigm shift in how cancer is diagnosed, treated, and managed. AI has already demonstrated immense potential in enhancing diagnostic accuracy and workflow efficiency, thus providing vital support to precision medicine. Through its capacity to automate the analysis of histopathological images, AI has not only contributed to faster diagnostics but has also ensured higher consistency and reduced intra- and inter-observer variability. As AI technology continues to evolve, its role in cancer pathology will expand, creating new opportunities for early detection, real-time diagnostics, and more personalized treatment strategies. AI’s strength lies in its ability to process vast amounts of data quickly and accurately, identifying complex patterns that may be challenging for human pathologists to detect. For example, AI algorithms have been shown to match or even exceed human performance in detecting various types of cancer, such as breast cancer, lung cancer, and melanoma, and in predicting treatment outcomes.[10,24] However, despite these advancements, the successful implementation of AI in clinical practice hinges on addressing several key challenges, such as data privacy, algorithm transparency, and regulatory compliance.
Moreover, the future of AI in cancer pathology is likely to be shaped by the continued development of XAI, which will increase trust among pathologists and clinicians by providing clear, interpretable outputs. Collaboration between AI systems and human pathologists will be essential, as AI is not intended to replace human expertise but to enhance it, enabling pathologists to focus on more complex cases and improve patient outcomes.[72] In addition, the use of AI in multi-modal data integration – combining histopathological images with genomic, clinical, and radiological data – will provide a more holistic view of each patient’s cancer, supporting the shift toward personalized oncology.[64]
Furthermore, AI-driven telepathology and remote diagnostics are expected to play a critical role in reducing healthcare disparities by providing high-quality diagnostic services to underserved and remote areas.[79] As AI becomes more accessible and its deployment costs decrease, these technologies could be scaled globally, democratizing access to advanced cancer diagnostics and improving outcomes for patients worldwide.
However, for AI to reach its full potential in cancer pathology, several hurdles must still be overcome. Ensuring the reliability and generalizability of AI models across diverse populations and healthcare settings is crucial to avoid the perpetuation of bias and inequity in healthcare.[71] Likewise, the ethical implications of AI must be carefully considered, ensuring that human pathologists remain in control of clinical decision-making and that patients receive the most personalized, safe, and effective care possible.
SUMMARY
AI holds immense promise in revolutionizing cancer pathology by improving diagnostic accuracy, streamlining workflows, and enabling personalized treatment strategies. While AI’s capabilities in automating tasks such as tumor detection and prognosis prediction are clear, the integration of AI into clinical practice must be approached carefully to overcome challenges related to data quality, model interpretability, and regulatory compliance. The future of AI in cancer pathology depends on the continued development of AI models that are both accurate and transparent, as well as on establishing clear guidelines and frameworks to ensure safe, equitable, and effective use in clinical settings. With concerted efforts from researchers, clinicians, and regulatory bodies, AI has the potential to transform the landscape of cancer diagnosis and treatment, ultimately improving patient outcomes on a global scale.
AVAILABILITY OF DATA AND MATERIALS
All data supporting the findings of this study are available from the corresponding author upon reasonable request.
ABBREVIATIONS
AI: Artificial intelligence
CNNs: Convolutional neural networks.
DL: Deep learning
Grad-CAM: Gradient-weighted Class Activation Mapping
HER2 score: Human epidermal growth factor receptor 2 score
IHC: Immunohistochemistry
ML: Machine learning
MSI: Microsatellite instability
TILs: Tumor-infiltrating lymphocytes
WSI: Whole-slide imaging
XAI: Explainable AI
ACKNOWLEDGMENT
Not applicable.
AUTHOR CONTRIBUTIONS
BC, DMS and JLW: Contributed to the conceptualization and design of the study; JLW and TW: Wrote the original draft manuscript; JLW and RH: Reviewed and edited the manuscript. All authors have read and agreed to the published version of the manuscript. All authors meet ICMJE authorship requirements.
ETHICS APPROVAL AND CONSENT TO PARTICIPATE
Ethical approval and consent to participate is not required as this article does not contain any studies on animals or patient, performed by any of the authors.
CONFLICT OF INTEREST
The authors declare no conflict of interest.
EDITORIAL/PEER REVIEW
To ensure the integrity and highest quality of CytoJournal publications, the review process of this manuscript was conducted under a double-blind model (authors are blinded for reviewers and vice versa) through an automatic online system.
FUNDING: This work was supported by the Medical and Health Science and Technology Development Project of Shandong, China (202411000466), Shandong medical staff science and technology innovation plan project (SDYWZGKCJHLH2023079), Shandong Provincial Natural Science Foundation (ZR2024QC075), and the Key Research and Development Plan of Jining (2024YXNS142), Shandong, China.
References
- Evidence for underuse of effective medical services around the world. Lancet. 2017;390:169-77.
- [CrossRef] [PubMed] [Google Scholar]
- Building cross-country networks for laboratory capacity and improvement. Clin Lab Med. 2018;38:119-30.
- [CrossRef] [PubMed] [Google Scholar]
- Artificial intelligence in digital pathology-new tools for diagnosis and precision oncology. Nat Rev Clin Oncol. 2019;16:703-15.
- [CrossRef] [PubMed] [Google Scholar]
- Going deep in medical image analysis: Concepts, methods, challenges, and future directions. IEEE Access. 2019;7:99540-72.
- [CrossRef] [Google Scholar]
- Machine learning methods for histopathological image analysis. Comput Struct Biotechnol J. 2018;16:34-42.
- [CrossRef] [PubMed] [Google Scholar]
- Pan-cancer image-based detection of clinically actionable genetic alterations. Nat Cancer. 2020;1:789-99.
- [CrossRef] [PubMed] [Google Scholar]
- AI-driven precision medicine: Transforming personalized cancer treatment. J AI Powered Med Innov. 2024;2:10-21.
- [CrossRef] [Google Scholar]
- Deep learning in histopathology: The path to the clinic. Nat Med. 2021;27:775-4.
- [CrossRef] [PubMed] [Google Scholar]
- Deep medicine: How artificial intelligence can make healthcare human again. 2019. United Kingdom: Hachette; Available from: https://books.google.com.hk/books?hl=zh-CN&lr=&id=_EFlDwAAQBAJ&oi=fnd&pg=PT8&dq=Topol+E.+Deep+medicine:+How+artificial+intelligence+can+make+healthcare+human+again.+United+Kingdom:+Hachette%3B+2019.&ots=BJ4yoW1BeX&sig=4mxQz014W8ds0pD51LTkNMWEJbo&redir_esc=y#v=onepage&q=Topol%20E.%20Deep%20medicine%3A%20How%20artificial%20intelligence%20can%20make%20healthcare%20human%20again.%20United%20Kingdom%3A%20Hachette%3B%202019.&f=false [Last accessed on 2024 Dec 23]
- [Google Scholar]
- Dermatologist-level classification of skin cancer with deep neural networks. Nature. 2017;542:115-8.
- [CrossRef] [PubMed] [Google Scholar]
- Implementation of digital pathology and artificial intelligence in routine pathology practice. Lab Invest. 2024;104:102111.
- [CrossRef] [PubMed] [Google Scholar]
- Mitosis detection in breast cancer histology images with deep neural networks. Medical image computing and computer-assisted intervention-MICCAI 2013 16th International Conference In: Nagoya, Japan, 2013, Proceedings, Part II 16. Germany: Springer; 2013. p. :411-8.
- [CrossRef] [PubMed] [Google Scholar]
- Errors in pathology and laboratory medicine: Consequences and prevention. J Surg Oncol. 2004;88:161-81.
- [CrossRef] [PubMed] [Google Scholar]
- Artificial intelligence in healthcare: Past, present and future. Stroke Vasc Neurol. 2017;2:e000101.
- [CrossRef] [PubMed] [Google Scholar]
- Pathologiststus LJ, Nelson HD, Onega T:n GM, Pepe MS, Reisch LM, ral netwos: Observer accuracy and reproducibility study. Br Med J. 2017;357:j2813.
- [CrossRef] [PubMed] [Google Scholar]
- Artificial intelligence in cancer imaging: Clinical challenges and applications. CA Cancer J Clin. 2019;69:127-57.
- [CrossRef] [PubMed] [Google Scholar]
- Artificial intelligence algorithms and their current role in the identification and comparison of gleason patterns in prostate cancer histopathology: A comprehensive review. Diagnostics. 2024;14:2127.
- [CrossRef] [PubMed] [Google Scholar]
- Artificial intelligence for the identification of biomarkers in cancer prevention and diagnosis: Advances and perspectives. Rev Brasil Cancerol. 2024;70:e254692.
- [CrossRef] [Google Scholar]
- Artificial intelligence in histopathology: Enhancing cancer research and clinical oncology. Nat Cancer. 2022;3:1026-38.
- [CrossRef] [PubMed] [Google Scholar]
- Artificial intelligence (AI) in medicine, current applications and future role with special emphasis on its potential and promise in pathology: Present and future impact, obstacles including costs and acceptance among pathologists, practical and philosophical considerations. A comprehensive review. Diagn Pathol. 2021;16:1-16.
- [CrossRef] [PubMed] [Google Scholar]
- Relevance of deep learning to facilitate the diagnosis of HER2 status in breast cancer. Sci Rep. 2017;7:45938.
- [CrossRef] [PubMed] [Google Scholar]
- Deep learning based tissue analysis predicts outcome in colorectal cancer. Sci Rep. 2018;8:3395.
- [CrossRef] [PubMed] [Google Scholar]
- Applications of artificial intelligence in breast pathology. Arch Pathol Lab Med. 2023;147:1003-13.
- [CrossRef] [PubMed] [Google Scholar]
- Classification and mutation prediction from non-small cell lung cancer histopathology images using deep learning. Nat Med. 2018;24:1559-67.
- [CrossRef] [PubMed] [Google Scholar]
- Medical ai for early detection of lung cancer: A survey. arXiv preprint arXiv:241014769 2024
- [Google Scholar]
- The application of artificial intelligence in lung cancer: A narrative review. Transl Cancer Res. 2021;10:2478.
- [CrossRef] [PubMed] [Google Scholar]
- The current issues and future perspective of artificial intelligence for developing new treatment strategy in non-small cell lung cancer: Harmonization of molecular cancer biology and artificial intelligence. Cancer Cell Int. 2021;21:454.
- [CrossRef] [PubMed] [Google Scholar]
- Deep-learning-based predictive imaging biomarker model for EGFR mutation status in non-small cell lung cancer from CT imaging. Cancers. 2024;16:1130.
- [CrossRef] [PubMed] [Google Scholar]
- Towards machine learning-aided lung cancer clinical routines: Approaches and open challenges. J Pers Med. 2022;12:480.
- [CrossRef] [PubMed] [Google Scholar]
- Decoding tumour phenotype by noninvasive imaging using a quantitative radiomics approach. Nat Commun. 2014;5:4006.
- [CrossRef] [PubMed] [Google Scholar]
- Development and validation of a deep learning algorithm for improving Gleason scoring of prostate cancer. NPJ Digit Med. 2019;2:48.
- [CrossRef] [PubMed] [Google Scholar]
- Automated Gleason grading of prostate cancer tissue microarrays via deep learning. Sci Rep. 2018;8:12054.
- [CrossRef] [PubMed] [Google Scholar]
- High-accuracy prostate cancer pathology using deep learning. Nat Mach Intell. 2020;2:411-8.
- [CrossRef] [Google Scholar]
- Pathologist-level grading of prostate biopsies with artificial intelligence. arXiv preprint arXiv:190701368 2019
- [Google Scholar]
- Evaluating the microsatellite instability of colorectal cancer based on multimodal deep learning integrating histopathological and molecular data. Front Oncol. 2022;12:925079.
- [CrossRef] [PubMed] [Google Scholar]
- Utility of artificial intelligence with deep learning of hematoxylin and eosin-stained whole slide images to predict lymph node metastasis in T1 colorectal cancer using endoscopically resected specimens; prediction of lymph node metastasis in T1 colorectal cancer. J Gastroenterol. 2022;57:654-66.
- [CrossRef] [PubMed] [Google Scholar]
- Melanoma clinical decision support system: An artificial intelligence-based tool to diagnose and predict disease outcome in early-stage melanoma patients. Cancers. 2023;15:2174.
- [CrossRef] [PubMed] [Google Scholar]
- Evaluation of melanoma thickness with clinical close-up and dermoscopic images using a convolutional neural network. Acta Derm Venereol. 2022;102:adv00790.
- [CrossRef] [PubMed] [Google Scholar]
- Artificial intelligence-assisted metastasis and prognosis model for patients with nodular melanoma. Plos One. 2024;19:e0305468.
- [CrossRef] [PubMed] [Google Scholar]
- Diagnostic outcomes of esophageal cancer by artificial intelligence using convolutional neural networks. Gastrointest Endosc. 2019;89:25-32.
- [CrossRef] [PubMed] [Google Scholar]
- Application of artificial intelligence for improving early detection and prediction of therapeutic outcomes for gastric cancer in the era of precision oncology. Semin Cancer Biol. 2023;93:83-96.
- [CrossRef] [PubMed] [Google Scholar]
- Landscape of innovative methods for early diagnosis of gastric cancer: A systematic review. Diagnostics. 2023;13:3608.
- [CrossRef] [PubMed] [Google Scholar]
- The value of artificial intelligence-assisted technology in HER2 assessmentof gastric cancer patients receiving neoadjuvant chemotherapy. China Oncol. 2023;33:377-87.
- [Google Scholar]
- A predictive model for treatment response in patients with locally advanced esophageal squamous cell carcinoma after concurrent chemoradiotherapy: Based on SUVmean and NLR. BMC Cancer. 2020;20:1-11.
- [CrossRef] [Google Scholar]
- Machine learning to predict curative multidisciplinary team treatment decisions in oesophageal cancer. Eur J Surg Oncol. 2023;49:106986.
- [CrossRef] [PubMed] [Google Scholar]
- Methodological quality of machine learning-based quantitative imaging analysis studies in esophageal cancer: A systematic review of clinical outcome prediction after concurrent chemoradiotherapy. Eur J Nucl Med Mol Imaging. 2022;49:2462-81.
- [CrossRef] [PubMed] [Google Scholar]
- Artificial intelligence in neuro-oncology: Advances and challenges in brain tumor diagnosis, prognosis, and precision treatment. NPJ Precis Oncol. 2024;8:80.
- [CrossRef] [PubMed] [Google Scholar]
- Emerging applications of artificial intelligence in neuro-oncology. Radiology. 2019;290:607-18.
- [CrossRef] [PubMed] [Google Scholar]
- AI-based glioma grading for a trustworthy diagnosis: An analytical pipeline for improved reliability. Cancers. 2023;15:3369.
- [CrossRef] [PubMed] [Google Scholar]
- Synergies of AI and smart technology: Revolutionizing cancer medicine, vaccine development, and patient care. Int J Soc Human Life Sci. 2023;1:10-8.
- [Google Scholar]
- The role of deep learning-based survival model in improving survival prediction of patients with glioblastoma. Cancer Med. 2021;10:7048-59.
- [CrossRef] [PubMed] [Google Scholar]
- U-Net: Convolutional networks for biomedical image segmentation In: Medical image computing and computer-assisted intervention. Cham: Springer; 2015. p. :234-41.
- [CrossRef] [Google Scholar]
- Preparing data for artificial intelligence in pathology with clinical-grade performance. Diagnostics. 2023;13:3115.
- [CrossRef] [PubMed] [Google Scholar]
- Artificial intelligence for solid tumour diagnosis in digital pathology. Br J Pharmacol. 2021;178:4291-315.
- [CrossRef] [PubMed] [Google Scholar]
- Minimum resolution requirements of digital pathology images for accurate classification. Med Image Anal. 2023;89:102891.
- [CrossRef] [PubMed] [Google Scholar]
- A survey on artificial intelligence in histopathology image analysis. Wiley Interdiscip Rev Data Mining Knowl Discov. 2022;12:e1474.
- [CrossRef] [Google Scholar]
- Embracing imperfect datasets: A review of deep learning solutions for medical image segmentation. Med Image Anal. 2020;63:101693.
- [CrossRef] [PubMed] [Google Scholar]
- Deep learning based active learning technique for data annotation and improve the overall performance of classification models. Expert Syst Applic. 2023;228:120391.
- [CrossRef] [Google Scholar]
- Introduction to digital image analysis in whole-slide imaging: A white paper from the digital pathology association. J Pathol Inform. 2019;10:9.
- [CrossRef] [PubMed] [Google Scholar]
- A survey on transfer learning. IEEE Trans Knowl Data Eng. 2010;22:1345-59.
- [CrossRef] [Google Scholar]
- A survey on image data augmentation for deep learning. J Big Data. 2019;6:60.
- [CrossRef] [Google Scholar]
- Clinical-grade computational pathology using weakly supervised deep learning on whole slide images. Nat Med. 2019;25:1301-9.
- [CrossRef] [PubMed] [Google Scholar]
- Grad-CAM: Visual explanations from deep networks via gradient-based localization. In IEEE International conference on computer vision 2017:618-26. Available from: http://dx.doi.org/10.1109/iccv.2017.74
- [CrossRef] [Google Scholar]
- Predicting cancer outcomes from histology and genomics using convolutional networks. Proc Natl Acad Sci U S A. 2018;115:E2970-9.
- [CrossRef] [PubMed] [Google Scholar]
- Secure, privacy-preserving and federated machine learning in medical imaging. Nat Mach Intell. 2020;2:305-11.
- [CrossRef] [Google Scholar]
- Exploring large-scale public medical image datasets. Acad Radiol. 2020;27:106-12.
- [CrossRef] [PubMed] [Google Scholar]
- A survey on explainable artificial intelligence (xai): Toward medical xai. IEEE Trans Neural Netw Learn Syst. 2020;32:4793-813.
- [CrossRef] [PubMed] [Google Scholar]
- Methods for interpreting and understanding deep neural networks. Digit Sig Process. 2018;73:1-15.
- [CrossRef] [Google Scholar]
- Ethical and legal challenges of artificial intelligence-driven healthcare In: Artificial intelligence in healthcare. Netherlands: Elsevier; 2020. p. :295-336.
- [CrossRef] [Google Scholar]
- Medical Malpractice and black-box medicine In: Glenn Cohen I, Lynch HF, Vayena E, Gasser U, eds. Big data, health law, and bioethics. University of Michigan Law School, Public Law Research Paper No 536. Cambridge, UK: Cambridge University Press; 2018. Available from: https://ssrn.com/abstract=2910417 [Last accessed on 2017 Feb 02]
- [Google Scholar]
- Addressing artificial intelligence bias in retinal diagnostics. Transl Vis Sci Technol. 2021;10:13.
- [CrossRef] [PubMed] [Google Scholar]
- High-performance medicine: The convergence of human and artificial intelligence. Nat Med. 2019;25:44-56.
- [CrossRef] [PubMed] [Google Scholar]
- The potential of AI in cancer care and research. Biochim Biophys Acta Rev Cancer. 2021;1876:188573.
- [CrossRef] [PubMed] [Google Scholar]
- The false hope of current approaches to explainable artificial intelligence in health care. Lancet Digit Health. 2021;3:e745-50.
- [CrossRef] [PubMed] [Google Scholar]
- Artificial intelligence in digital histopathology for predicting patient prognosis and treatment efficacy in breast cancer. Expert Rev Mol Diagn. 2024;24:363-77.
- [CrossRef] [PubMed] [Google Scholar]
- The Role of artificial intelligence on tumor boards: Perspectives from surgeons, medical oncologists and radiation oncologists. Curr Oncol. 2024;31:4984-5007.
- [CrossRef] [PubMed] [Google Scholar]
- Artificial intelligence-based breast cancer nodal metastasis detection: Insights into the black box for pathologists. Arch Pathol Lab Med. 2018;143:859-68.
- [CrossRef] [PubMed] [Google Scholar]
- AI-assisted detection of biomarkers by sensors and biosensors for early diagnosis and monitoring. Biosensors. 2024;14:356.
- [CrossRef] [PubMed] [Google Scholar]
- Wholeslide imaging equivalency and efficiency study: experience at a large academic center. Mod Pathol. 2019;32:916-28.
- [CrossRef] [PubMed] [Google Scholar]
- Towards a rigorous science of interpretable machine learning. 2017. arXiv Preprint. [Last accessed on 2024 Dec 23]
- [CrossRef] [Google Scholar]
- Lifelong machine learning: A paradigm for continuous learning. Front Computer Sci. 2017;11:359-61.
- [CrossRef] [Google Scholar]
- Methodologies for continuous, life-long machine learning for AI systems In: Artificial psychology: Psychological modeling and testing of AI systems. 2020. p. :129-38.
- [CrossRef] [Google Scholar]
- Feedback-based self-learning in large-scale conversational ai agents. AI Magazine. 2022;42:43-56.
- [CrossRef] [Google Scholar]
- DNA methylation-based classification of central nervous system tumours. Nature. 2018;555:469-74.
- [CrossRef] [PubMed] [Google Scholar]
- Opportunities and obstacles for deep learning in biology and medicine. J R Soc Interface. 2018;15:20170387.
- [CrossRef] [PubMed] [Google Scholar]
- Deep learning-based breast cancer classification through medical imaging modalities: State of the art and research challenges. Artif Intell Rev. 2020;53:1655-20.
- [CrossRef] [Google Scholar]